[ad_1]
Evolution trend of population distribution pattern in China
The changing trend of China’s population distribution pattern is closely tied to the facility agglomeration of urban industrial facilities, which significantly impacts population dynamics47. The accelerated pace of economic development and urbanization has made the influence of urban industrial facility agglomeration increasingly pronounced. This phenomenon leads to the concentration of industries in specific regions, such as the Yangtze River Delta, the Pearl River Delta, and the Beijing-Tianjin-Hebei region, resulting in distinct industrial facility agglomeration zones25. Moreover, the size of urban areas attracts more industrial investment and facility construction, creating a positive feedback loop between population growth and industrial development. Therefore, understanding the impact of urban industrial facility agglomeration is vital for comprehending the evolving trend of China’s population distribution pattern. Building upon this understanding, this paper offers a comprehensive analysis of China’s population distribution pattern changing in urban industrial facility agglomeration. The findings of this study provide a scientific basis for formulating policies that can promote regional economic development7,8.
Population spatial distribution characteristics
The Hu Line has played a crucial role in identifying China’s population distribution pattern, dividing the country into the southeast and northwest regions. This line serves as a boundary, revealing significant disparities in population distribution. The densely populated areas are primarily located in the east, while the west remains sparsely populated (Fig. 2). Over the years, China has witnessed substantial population migration, predominantly occurring in the southeast. Meanwhile, the northwest has experienced higher population growth due to higher birth rates and lower emigration rates. The proportion of the population in the northwest has gradually increased from 5.77% in 1982 to 6.5% in 2020. The seventh national population census in 2020 confirms the influence of natural geographical environments on population distribution stability. From 2015 to 2021, areas with a population density of over 1000 person/km2 were predominantly found in the southeast, while only Lanzhou, Yinchuan, and Shihezi in the northwest had a density of over 300 person/km2.
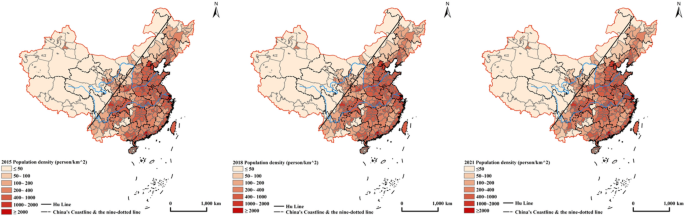
Distribution pattern of population density in China from 2015 to 2021. This map displays the distribution pattern of population density in China from 2015 to 2021. Generated using ArcGIS Desktop 10.8 (ArcMap 10.8, Release Version 10.8, Product Version 10.7.0.10450).
The southeast side of the Hu Line, encompassing coastal areas such as Guangdong, Zhejiang, and Jiangsu, exhibits higher population density. In contrast, the northwest side, including Tibet, Xinjiang, and Qinghai, has a lower population density due to climatic and natural conditions. The eastern coastal areas have experienced higher urbanization rates, reflecting the process of population migration from rural to urban areas. The eastern region’s higher urbanization rate has contributed to its relatively higher economic and social development. However, from 2015 to 2021, the aging population issue worsened, particularly in the eastern region, due to declining fertility rates and increasing life expectancy48. Population mobility remains high in the western region, driven by disparities in economic development between the western and eastern coastal areas49. Many young individuals from the western region seek better opportunities in the eastern coastal cities, resulting in a continuous decrease in the western region’s population.
From 2015 to 2021, China experienced continued large-scale population migration, with some notable shifts compared to previous years. Urbanization, rising production costs, stricter management policies for migrant workers, and evolving economic and demographic structures have influenced population migration patterns. These changes have affected the prevalence of rural migrant workers, prompting some to return to their hometowns or seek opportunities elsewhere. In certain areas, stricter management policies have constrained population mobility. Furthermore, rapid economic growth and urbanization have led to aging populations and declining population figures in some cities. Conversely, economic development in the western regions has attracted population inflows. These factors collectively contribute to the transformation of population migration patterns.
Characteristics of population distribution equilibrium changes
The Gini coefficient is a practical indicator widely used to assess population distribution inequality in China. This study utilizes rasterized population data from 2000 to 2021 to calculate the Gini coefficient, revealing minor fluctuations but an overall upward trend (Fig. 3). This indicates a growing imbalance in population distribution. Efforts to foster balanced distribution include developing the Western regions’ economy, improving infrastructure connectivity, and encouraging regional coordinated development. Addressing this imbalance requires persistent implementation of effective policies.
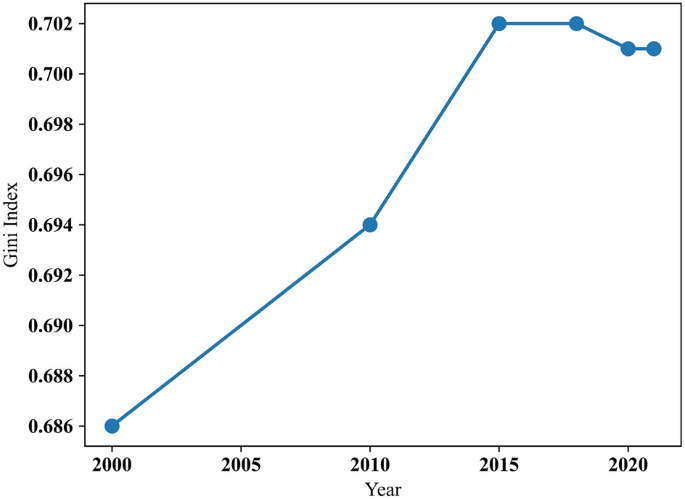
Gini coefficient of population distribution in China from 2000 to 2021.
Regional disparities in economic development have intensified population distribution imbalances. Rapid economic growth in the eastern regions attracted migrants while lagging development in the west led to population outflows. Variations in natural geographical conditions also contribute to these imbalances, with the east offering favorable conditions and the west experiencing lower population densities due to limiting factors.
Analyzing specific periods, the Gini coefficient increased between 2000 and 2010 due to rapid economic development and urbanization. Between 2010 and 2015, the growth slowed as the government supported western and central-western regions, curbing inequality. From 2015 to 2018, stability was observed due to regional development efforts. Between 2018 and 2021, a slight decrease occurred as the government focused on coordinated development, and inland cities and western regions experienced growth.
The Gini coefficient ranges from 0 to 1, with 0 indicating equitable distribution and 1 representing uneven distribution. The Lorenz curve visually represents distributional inequality, with the Gini coefficient derived from it. China’s Lorenz curve for population distribution demonstrates significant inequality driven by economic development, urbanization, and migration. The curve shows concentration in economically developed eastern coastal regions and large cities.
The Gini coefficient decreased slightly by 2018 and 2021, reflecting a modest reduction in population distribution imbalance50. Efforts to bolster regional coordination and develop central and western regions contributed to this change. However, the imbalance persists, albeit with limited improvement. Economic transition and ongoing regional development strategies contribute to the decreasing trend51. The specific Lorenz curve for China’s population distribution is depicted in Fig. 4.
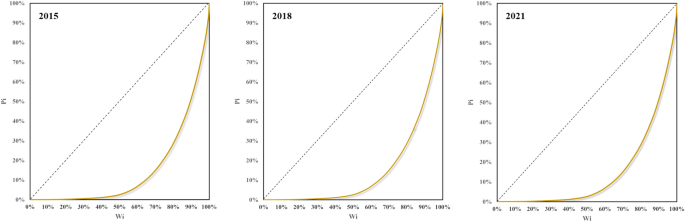
Lorenz curve of population distribution in China from 2015 to 2021.
Spatial analysis of urban industrial facility agglomeration
Amidst globalization and urbanization, urban industrial facility agglomeration has emerged as a critical driver of economic development and urban competitiveness. Industrial facility agglomeration fosters communication and collaboration among industries, enhancing production efficiency and stimulating employment, innovation, and regional development. To uncover the intrinsic relationship between urban industrial facility agglomeration and urban population growth rates, this study utilizes spatial analysis methods to examine the influence of spatial distribution characteristics and agglomeration degrees of 12 industry types on urban population growth rates.
In conjunction with POI data, this paper employs a kernel density algorithm to characterize these industry types and analyze the spatial distribution patterns of various urban industries across China, as depicted in Fig. 5. Observations from the 3D kernel density maps of the 12 industries in 2015 and 2021 reveal the following trends.
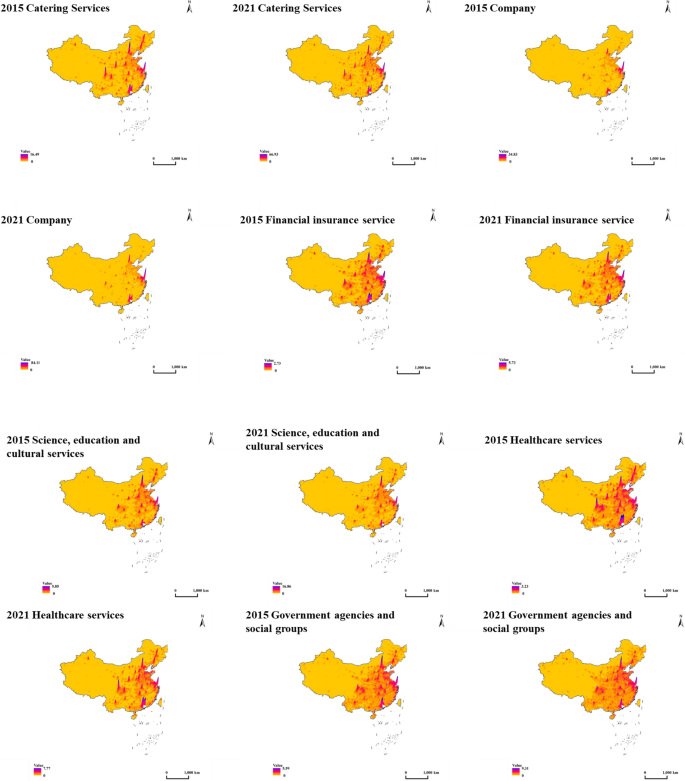
3D kernel density map of industrial facilities in each city in 2015 and 2021. A 3D kernel density map representing the spatial distribution of industrial facilities in various cities for the years 2015 and 2021. Created using ArcGIS Desktop 10.8 (ArcScene 10.8, Release Version 10.8, Product Version 10.7.0.10450).
To sum up, between 2015 and 2021, the high-density regions across various industries were primarily concentrated in the Beijing-Tianjin-Hebei, Yangtze River Delta, and Pearl River Delta areas. These regions play a crucial role in China’s economic development and are characterized by high output value and population density. Beijing and Shanghai consistently emerged as the top industry categories’ top performers, reflecting their positions as China’s political and economic hubs52. Furthermore, cities like Chengdu and Chongqing highly competed in specific industry sectors.
Global autocorrelation analysis
In this section, we utilized the Global Moran’s I index to calculate the spatial autocorrelation of 12 types of industrial facilities across China in 2015, 2018, and 2021. Each industry type exhibited significant spatial autocorrelation at all three time points, indicating that industrial facilities in each region were highly correlated with adjacent geographic units. The changes in the Global Moran’s I value from 2015 to 2021 are presented in Table 2.
The spatial autocorrelation of the 12 types of industrial facilities displayed significant spatial clustering at all three time points. Notably, the Company type had the strongest spatial correlation with Global Moran’s I values of 0.223 (in 2015), 0.237 (in 2018), and 0.231 (in 2021). This suggests that the spatial distribution of Company type industrial facilities exhibited the strongest correlation across China during the study period. Additionally, Famous tourist sites, Shopping service, and Financial insurance service types also exhibited relatively high Global Moran’s I value of 0.167 (in 2021), 0.199 (in 2021), and 0.167 (in 2021), respectively. The z-values of all industries at the three-time points were significantly greater than zero, and the p-values were zero, indicating that the Global Moran’s I was highly significant, and spatial autocorrelation was significant across these industries53. These results demonstrate that the spatial distribution of the 12 types of industrial facilities in China exhibited a certain degree of clustering during the study period, which may be attributed to the economic development level, industrial structure, and policy orientation of different regions in China54.
By comparing the Global Moran’s I value of 12 industries across China in 2015, 2018, and 2021, this article can analyze the changing trends in spatial autocorrelation. Catering Services showed a consistent year-on-year increase at all three time points, indicating a continuous rise in the spatial agglomeration of catering facilities. From 2015 to 2018, Famous tourist sites displayed a decreasing trend but slightly rebounded from 2018 to 2021, suggesting a fluctuation in the spatial agglomeration of tourist attractions yet remaining relatively stable overall. The Global Moran’s I value of Company facilities increased from 2015 to 2021, indicating an overall increase in the spatial agglomeration of company facilities55. Similarly, Shopping service, Financial insurance service, Science, education and cultural services, Domestic services, Sports and Leisure Services, Healthcare services, and Government agencies and social groups all showed an increasing trend in their Global Moran’s I value from 2015 to 2021, indicating an overall increase in the spatial agglomeration of facilities in these industries56. However, Business residence showed an overall decreasing trend from 2015 to 2021, indicating a reduction in the spatial agglomeration of commercial and residential facilities. Accommodation services also decreased from 2015 to 2021, indicating decreased spatial agglomeration of accommodation facilities.
Local autocorrelation analysis
This section uses the Local Moran’s I index to compute the spatial autocorrelation of 12 industrial facilities across China and examine the interrelationships among various industry types among administrative units at or above the prefectural level. The LISA map is an indicator that assesses the degree of similarity and dissimilarity and its significance between spatial unit attributes and neighboring units. LISA clustering maps depict four distinct types of spatial autocorrelation relationships: High-High (H–H), Low–Low (L–L), Low–High (L–H), and High–Low (H–L). H–H type represents a high level of industrial facility agglomeration in the study area and its adjacent regions. In contrast, the L–L type indicates a low level of industrial facility agglomeration in the study area and its surrounding areas. The L–H type suggests that the industrial facility agglomeration level is low in the study area but high in its surrounding areas. In contrast, the H–L type indicates a high industrial facility agglomeration level in the study area but low in its surrounding areas. By calculating the Moran’s I value of industrial facility agglomeration distribution among administrative units at or above the prefectural level in 2015 and 2021 and drawing LISA clustering maps based on Z-test values (P = 0.05), readers can observe the changes and trends of industrial facility agglomeration as shown in Fig. 6. An in-depth analysis of data for the 12 types of industries reveals that the number of prefectural and higher-level units for each type of industry generally exhibits an increasing trend. Moreover, the Beijing-Tianjin-Hebei, the Yangtze River Delta, and the Pearl River Delta regions show high agglomeration levels in most industries. However, regions such as Tibet, Qinghai, Xinjiang, Gansu, southwest Inner Mongolia, southern Guangxi, and Hainan exhibit relatively low agglomeration levels in most industries. Further research into the factors that affect the spatial agglomeration of facilities in various industries, such as policy changes, regional development strategies, industrial structure adjustments, and population mobility, can provide targeted recommendations for urban planning, industrial policy development, and regional economic development.
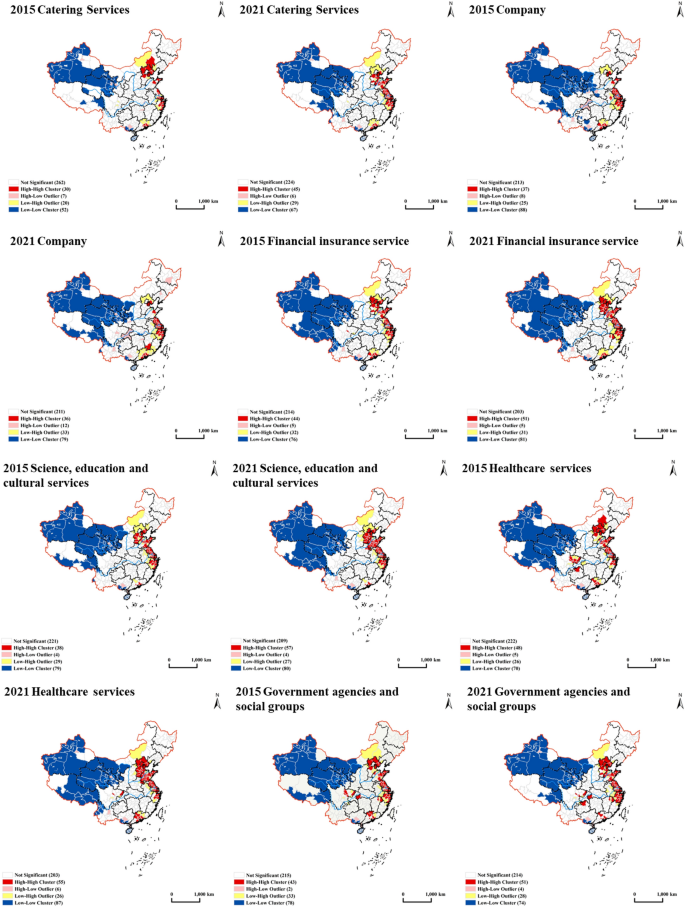
LISA map of industrial facility agglomeration in China in 2015 and 2021. This map visualizes the Local Indicators of Spatial Association for the agglomeration of industrial facilities in China during 2015 and 2021. Produced with ArcGIS Desktop 10.8 (ArcMap 10.8, Release Version 10.8, Product Version 10.7.0.10450).
In conclusion, the spatial autocorrelation relationships of various industries underwent significant changes between 2015 and 2021. In general, the number of H–H level prefectural and higher-level administrative units increased in the Beijing-Tianjin-Hebei, Yangtze River Delta, and Pearl River Delta regions, indicating an increase in agglomeration. Conversely, the number of H–L level units is relatively small and mainly concentrated in central and northeastern China’s provincial capitals or central cities. The distribution of L–H level units is uneven among industries, primarily concentrated in the northern part of Hebei and southeastern Anhui. In contrast, the number of L–L level units is relatively high and mainly distributed in areas with lower economic development levels, such as Tibet, Qinghai, and Xinjiang. A comparative analysis of the 2015 and 2021 data shows that industry facility agglomeration tends to concentrate, particularly in economically developed regions. This trend is closely linked to policy support, infrastructure construction, and market demand. However, some relatively underdeveloped regions still face challenges in industrial development. Therefore, more support is necessary for these areas in the future to promote industry development and spatial optimization.
Spatial heterogeneity analysis of population distribution and industrial facility agglomeration
This study used the geographical detectors method to analyze the agglomeration of various industries and its impact on urban population growth rates. Table 3 presents the results of the factor detector for 2015 and 2021, including q-statistic values and p values. The findings reveal significant disparities in the influence of different factors on population growth rates between the two periods. Note that “A B C …” represents different types of POIs (A, B, C, …, L).
In 2015, Science, Education, and Cultural Services (F), Business Residence (G), and Financial Insurance Services (E) had a substantial impact on urban population growth rates (q = 0.217, q = 0.183, and q = 0.171, respectively, all with p < 0.001). This suggests that these factors played a significant role in shaping population growth in 2015. However, in 2021, Science, Education, and Cultural Services (F) and Government Agencies and Social Groups (K) emerged as the key influencers (q = 0.092 and q = 0.096, respectively, both with p < 0.001). These findings indicate that the factors influencing population growth rates have evolved over time, with Science, Education, and Cultural Services and Government Agencies and Social Groups gaining increasing importance.
It is worth noting that certain factors had a relatively low impact on population growth rates in both periods. For example, Domestic Services (H) had a q-statistic of 0.162 in 2015, which decreased to 0.055 in 2021. Similarly, Accommodation Services (L) had a q-statistic of 0.123 in 2015, further decreasing to 0.038 in 2021. These results suggest that these factors have a minor influence on urban population growth, with varying degrees of impact across different periods.
The analysis reveals a shift in the key factors influencing urban population growth rates over time. Government Agencies and Social Groups gained significance, while factors like Business Residence and Financial Insurance Services exhibited a weaker impact. Notably, Science, Education, and Cultural Services consistently strongly influenced both periods. This emphasizes the crucial role of education, scientific research, and government policies in shaping population distribution and industry facility agglomeration dynamics.
The evolution and influence of China’s industrial facility agglomeration on the shape of population distribution pattern
This study examines the impact of industrial facility agglomeration on population distribution patterns in China from 2015 to 2021. Using a linear regression model and data visualization techniques, the relationships between urban industrial facilities and population growth rates are analyzed57. The findings reveal linear relationships between industrial facility agglomerations and population growth rates, with variations in strength and direction between the two time periods (Fig. 7). Please note that the Population Growth Rate is calculated as follows: (End-of-Year Population − Beginning-of-Year Population)/Beginning-of-Year Population × 100%. In 2015, Financial Insurance Services had a significant impact on population growth rates. However, this influence weakened in 2021 due to the deceleration of overall population growth and the emergence of other influential factors. The correlation coefficients between industrial facility agglomerations and population growth rates shifted from positive to negative during this period, reflecting changes in China’s population policies and economic structure58. Notably, the relationships between Famous Tourist Sites, Science, Education and Cultural Services, Government Agencies and Social Groups, and population growth rates weakened in 2021, attributed to government initiatives promoting industrial transformation and upgrading59. Emerging industries, high-tech, and service sectors have gained more attractiveness, while traditional industrial facilities have comparatively diminished appeal.
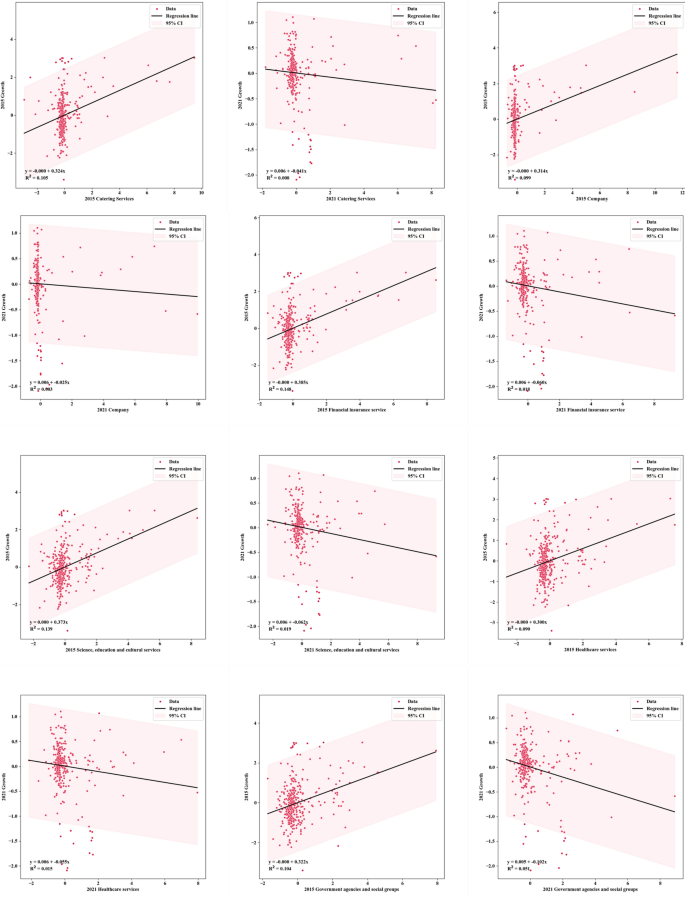
Scatter plot of China’s urban population growth rate and urban industrial facility agglomeration in 2015 and 2021.
This analysis examines the relationship between urban industrial facility agglomeration and urban population growth in different regions of China. The focus is on the Catering Services industry as a case study (Fig. 8), with supplementary materials providing information on other industries.
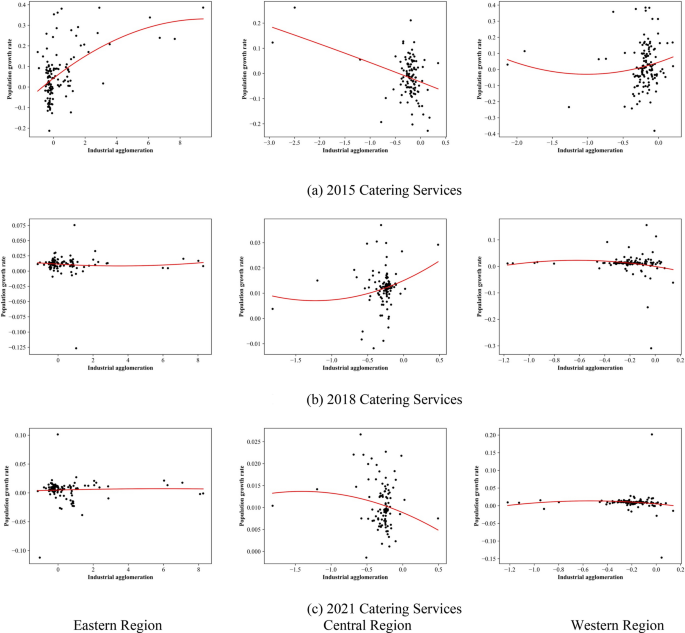
The relationship between industrial facility agglomeration and population growth by dividing China into eastern region, central region, and western region.
In 2015, the eastern region showed high industrial facility agglomeration in Catering Services, positively correlated with population growth. The central region had lower industrial facility agglomeration, negatively correlated with population growth. The western region had low industrial facility agglomeration, with an insignificant correlation to population growth. By 2018, the eastern region maintained high industrial facility agglomeration in Catering Services, but the correlation with population growth was unclear. The central region showed low industrial facility agglomeration, positively correlated with population growth. The western region maintained low industrial facility agglomeration, with an insignificant correlation to population growth. In 2021, the eastern region still had high industrial facility agglomeration in Catering Services, but the relationship with population growth was unclear. The central region had low industrial facility agglomeration, negatively correlated with population growth. The western region had low industrial facility agglomeration, with an insignificant correlation to population growth.
Overall, industrial facility agglomeration for other industries had specific characteristics. In the eastern region, agglomeration concentrated between (0, 2), while in the central and western regions, it concentrated between (− 0.5, 0). Various factors influenced these patterns:
Eastern Region Rapid economic growth and urbanization led to industry saturation, resulting in small changes in agglomeration. Urbanization and population aging slowed population growth and weakened the correlation with agglomeration. Economic restructuring towards the service and innovation sectors is crucial for sustainable growth.
Central Region Lower economic development and social resources limited population growth compared to the eastern region. Attracting advantageous industries from the east can improve employment and economic development. Industries like Company, Science, Education, Business Residence, and Accommodation Services performed significantly.
Western Region Initially reliant on natural resource development, the region faced limitations and weakened agglomeration. Strengthening industrial diversification and fostering innovation-driven development is necessary. Industries like Famous Tourist Sites, Company, Financial Insurance Services, and Science, Education and Cultural Services performed remarkably. Focus on tourism, business support, financial services, and science, education, and culture investments can promote growth.
This study conducted a multiple regression analysis on the relationship between urban population growth rates and agglomerations of industrial facilities in China. Data from 2015, 2018, and 2021 were analyzed, and comprehensive results can be found in Table 4.
In 2015, after controlling for other factors, Financial Insurance Services agglomeration showed a significantly positive correlation with urban population growth rates. On the other hand, Business Residence and Accommodation Services agglomerations had significantly negative correlations. These findings suggest that Financial Insurance Services were attractive to urban populations in 2015, while Business Residence and Accommodation Services were less appealing. The regression model explained about 16.6% of the variation in population growth rates (R2 = 0.166).
In 2018, the regression results showed a significantly negative correlation between Company agglomeration and urban population growth rates. Financial Insurance Services and Science, Education, and Cultural Services agglomerations had significant positive and negative correlations. This indicates that Financial Insurance Services remained attractive to urban populations in 2018, while Companies and Science, Education, and Cultural Services were less appealing. The regression model explained about 4.7% of the variation in population growth rates (R2 = 0.047).
In 2021, Catering Services, Companies, Government Agencies, and Social Groups agglomerations were significantly correlated with urban population growth rates. Business Residence and Domestic Services agglomerations had significantly negative correlations. This suggests that Catering Services, Companies, Government Agencies, and Social Groups were highly appealing to urban populations in 2021, while Business Residence and Domestic Services were less enticing. The regression model explained about 5.3% of the variation in population growth rates (R2 = 0.053).
Over the study period, the relationships between population growth rates and agglomerations of industrial facilities in China’s administrative units experienced notable changes. Financial Insurance Services consistently attracted urban populations, while the appeal of other industries varied. This highlights the impact of industrial restructuring and transformation on population distribution patterns during China’s urban development. As China’s economy advances and its industrial structure evolves, high-value-added industries and service sectors will be crucial in shaping future urban population distribution. Therefore, the government must prioritize optimizing industrial facility layouts, promoting industrial restructuring and transformation, and fostering sustainable urban development to address these evolving population patterns.
[ad_2]
Source link